Integrity of Data in AI Models
When it comes to AI investment, ensuring the integrity of data used in AI models is crucial. Biased or inaccurate data can lead to unfair or discriminatory outcomes, which can have significant ethical implications. That’s why a robust data governance framework is essential in prioritizing data quality, transparency, and accountability.
Investors and stakeholders must be vigilant in ensuring that the data used to train AI models is accurate and free from bias. This means carefully vetting the sources of data and continuously monitoring and updating the data to ensure its integrity.
In a recent article on Potential Venture Capital in AI, it was highlighted how important it is for investors to understand the potential impact of biased or inaccurate data on AI models. The article emphasized the need for due diligence in scrutinizing the integrity of the data used in AI investment strategies.
Transparency and interpretability of algorithms also play a key role in maintaining the integrity of AI models. Investors should seek out AI solutions that offer clear explanations of how they arrive at their decisions, allowing for scrutiny and validation of their processes.
Furthermore, establishing accountability structures for AI applications is pivotal in addressing any ethical concerns related to data integrity. This involves clearly defining responsibilities and oversight mechanisms to ensure that any issues related to data integrity are swiftly addressed.
In conclusion, maintaining the integrity of data in AI models is a critical aspect of ethical AI investment. By prioritizing data quality, transparency, and accountability, investors can mitigate ethical risks associated with biased or inaccurate data. It’s vital for stakeholders to stay informed about the potential pitfalls related to data integrity in AI investment and take proactive measures to address them.
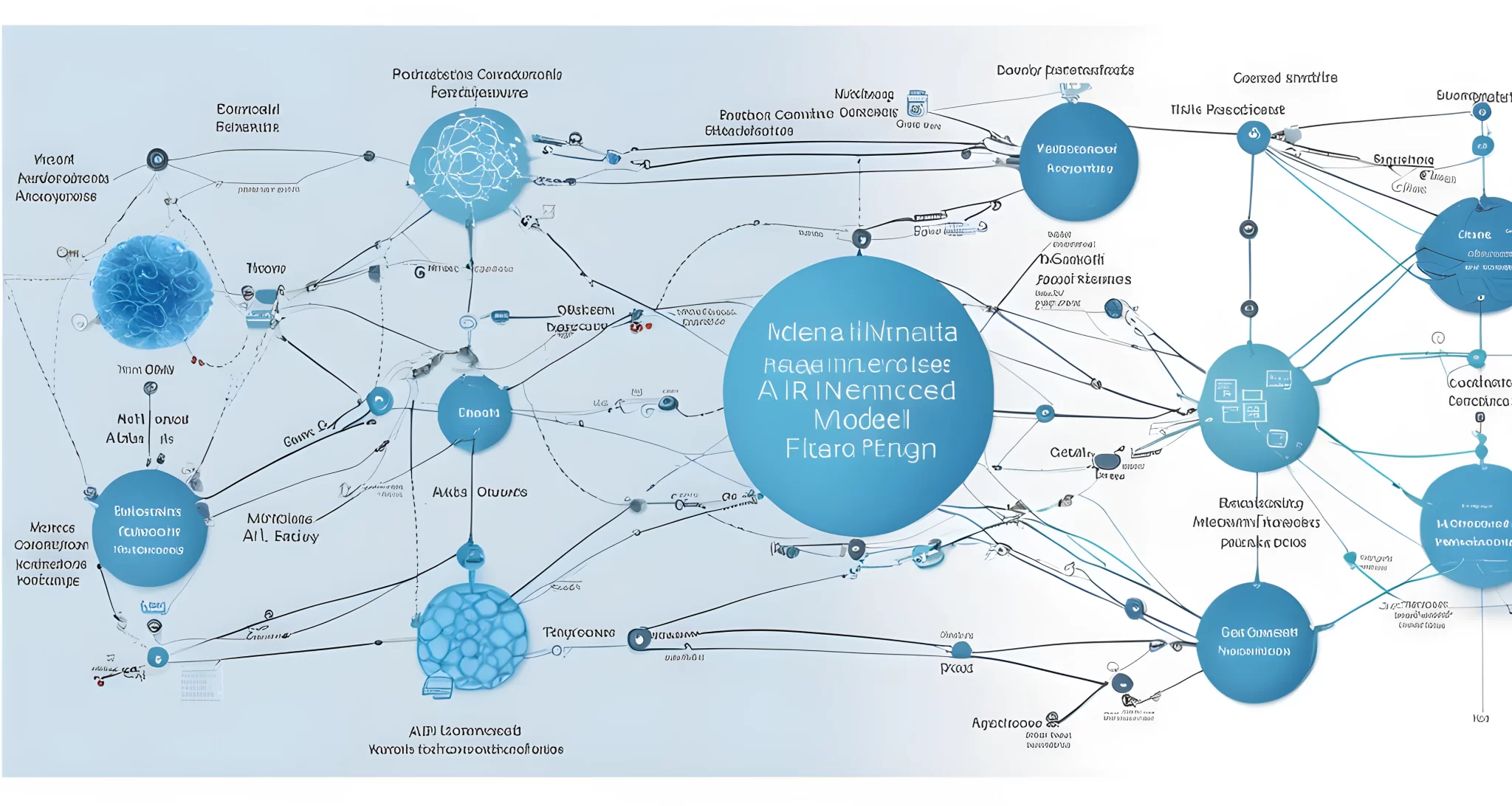
Accuracy and Validity of AI Models
When it comes to AI models in the investment management industry, ensuring accuracy and validity is paramount. These models are used to make predictions and inform important financial decisions, so any inaccuracies or biases could have significant consequences. Regular testing and review of AI models are necessary to ensure that they are performing as expected and evolving in a reliable manner.
One of the key considerations in ensuring the accuracy and validity of AI models is the integrity of the data used to train and test these models. This includes measures to identify and mitigate biases and inaccuracies in the data, as well as in the model’s training process. Without clean and reliable data, AI models can produce flawed results, leading to poor investment decisions.
Transparency and interpretability of algorithms also play a crucial role in ensuring the accuracy and validity of AI models. It is important for investment professionals to understand how these algorithms work and how they arrive at their conclusions. This allows for better oversight and validation of the model’s predictions.
In addition, accountability structures for AI applications are essential for maintaining the accuracy and validity of AI models. These structures ensure that there are clear lines of responsibility for the performance of AI models, as well as processes for addressing any issues that may arise.
To further mitigate risks associated with AI models, businesses can implement best practices for managing AI risks Businesses AI risk mitigation. These best practices include regular auditing of AI systems, establishing clear governance structures, and implementing robust testing processes.
In conclusion, ensuring the accuracy and validity of AI models is crucial for ethical decision-making in investment management. By prioritizing data integrity, transparency, interpretability, and accountability, businesses can mitigate risks associated with AI applications while making informed investment decisions.
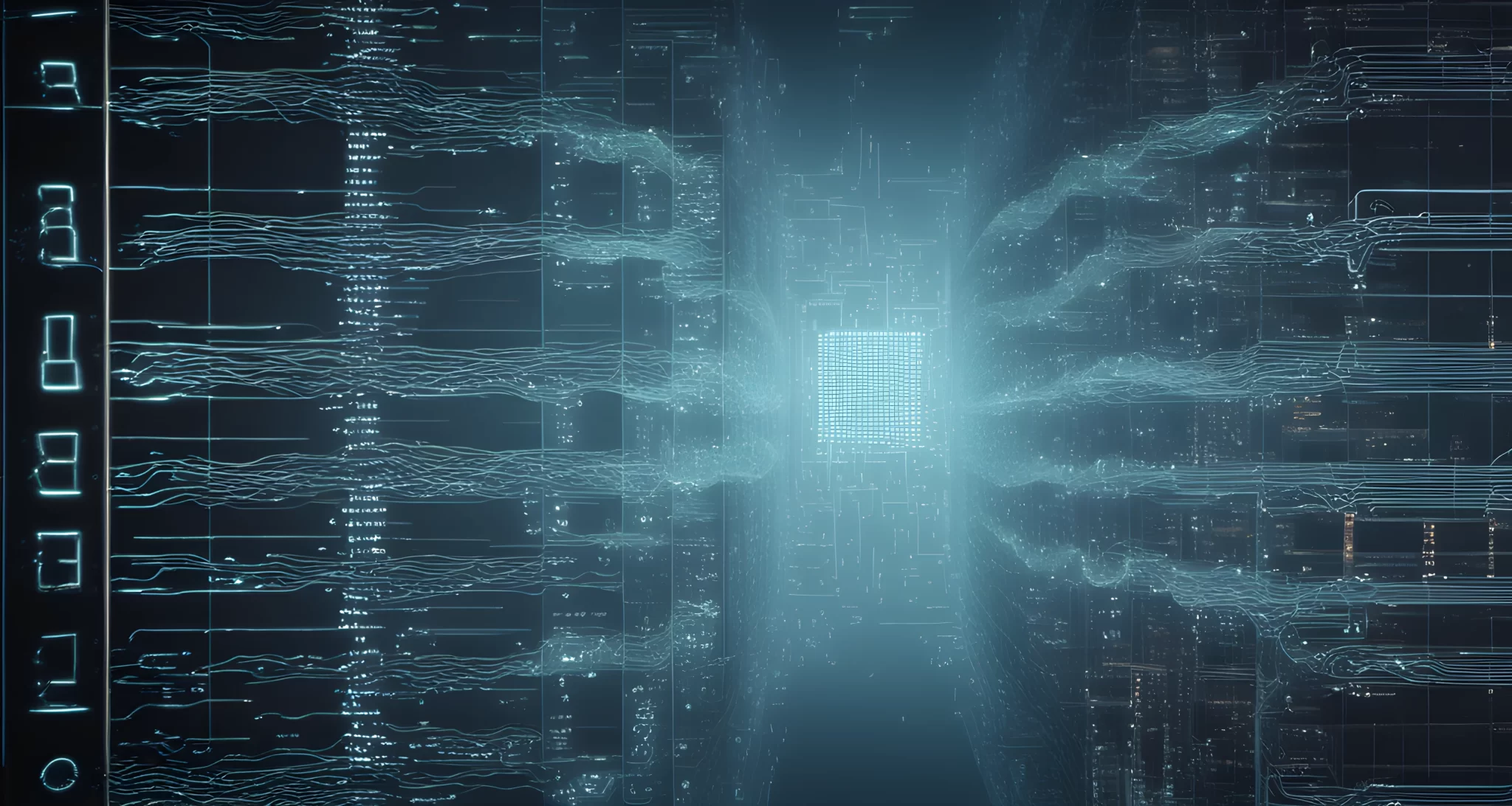
Transparency and Interpretability of Algorithms
Transparency and interpretability of algorithms are crucial components for ensuring the ethical and responsible use of AI in investment management. It is essential for stakeholders to understand how AI makes decisions and who is accountable for those decisions. Developing explainable AI is important for characterizing the fairness, accuracy, and potential bias of AI models. This helps in identifying and addressing any unintended consequences of AI decision-making.
One way to achieve transparency and interpretability is through the use of interpretable machine learning techniques, such as decision trees and linear models. These techniques provide a clear understanding of how the AI model arrives at its decisions, making it easier to identify any biases or errors. Additionally, ensuring that the data used to train AI models is transparent and free from biases is critical for maintaining the integrity of the models.
Moreover, accountability structures for AI applications should be established to hold individuals or organizations responsible for any unethical or biased decisions made by AI systems. This includes implementing processes for auditing, monitoring, and reporting on the performance of AI algorithms.
By addressing transparency and interpretability in AI algorithms, stakeholders can gain confidence in the ethical use of AI in investment management. This ultimately leads to more informed decision-making processes and builds trust with investors.
For more information on challenges in investment management, you can read our article on Overcoming Investment Hurdles.
In conclusion, ensuring transparency and interpretability of algorithms in AI investment management is essential for maintaining ethical standards and building trust with stakeholders. By implementing these principles, organizations can demonstrate a commitment to responsible AI practices and mitigate potential risks associated with biased or unfair decision-making.
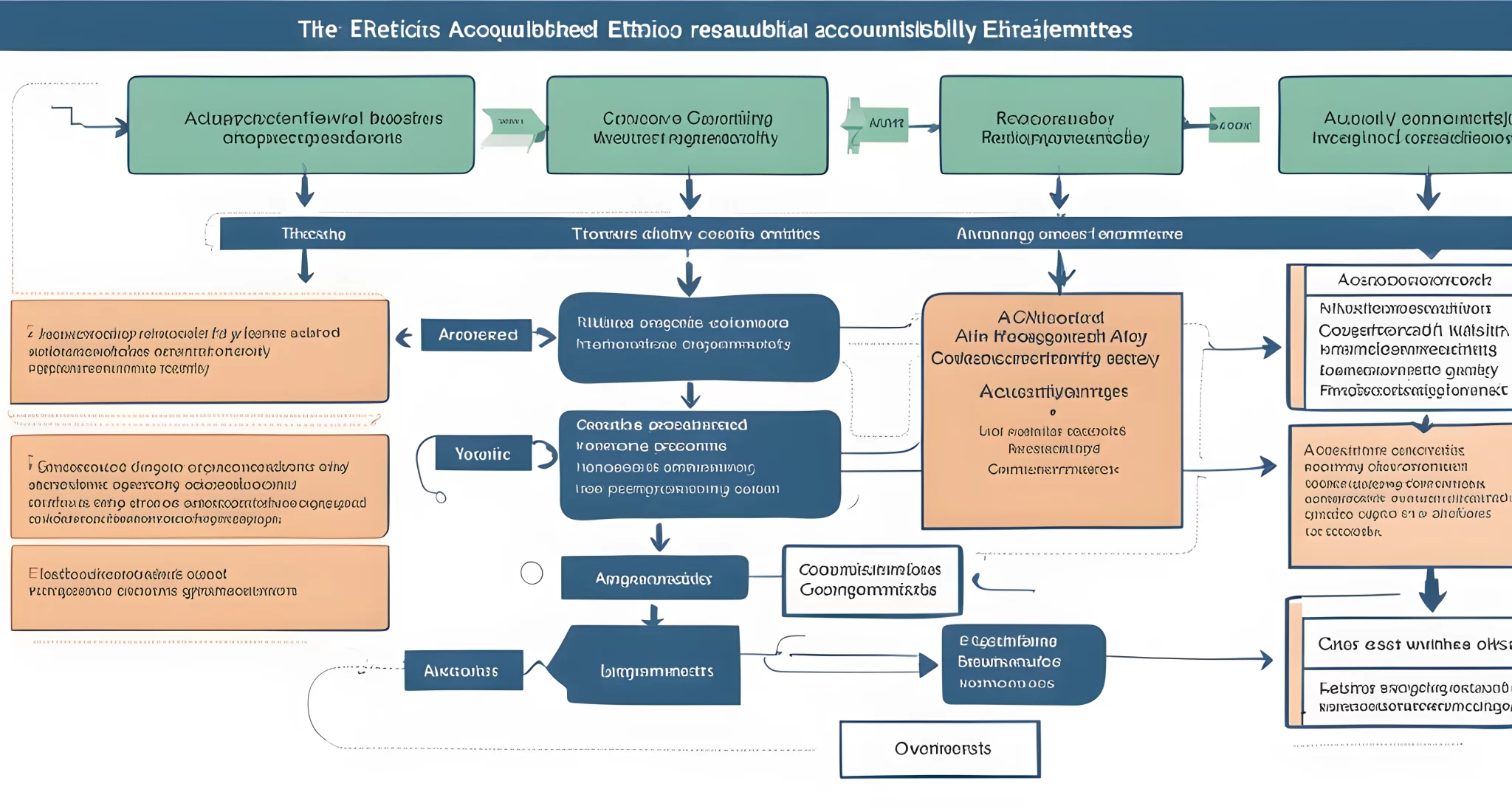
Accountability Structures for AI Applications
Establishing accountability structures is vital to ensure that AI applications serve the best interest of clients. It involves setting up mechanisms for addressing errors or harm caused by AI systems and ensuring that appropriate corrective actions can be taken. This is crucial in the financial services industry, where trust and reliability are paramount. According to AI’s Impact on Finance, AI is transforming financial services by providing new opportunities for efficiency and innovation, but it also comes with ethical challenges that must be addressed.
One of the key aspects of accountability structures for AI applications in investment management is defining clear roles and responsibilities for those involved in the development and deployment of AI. This includes not only the developers and data scientists but also compliance officers, risk managers, and senior leadership. Each stakeholder should understand their role in ensuring the ethical use of AI and be held accountable for their contributions.
Furthermore, accountability structures should include mechanisms for transparency and oversight. This means providing clear documentation of how AI models are developed, tested, and deployed, as well as establishing processes for ongoing monitoring and evaluation. In the event of errors or harmful outcomes, there should be a clear process for identifying the cause, taking corrective action, and communicating with affected parties.
In addition to internal accountability structures, external oversight may also be necessary. This could involve regulatory bodies or independent auditors who can provide an objective assessment of the ethical implications of AI applications in investment management.
Ultimately, accountability structures for AI applications are essential to building trust and confidence in the use of AI in investment management. By defining roles and responsibilities, promoting transparency, and establishing mechanisms for oversight, organizations can ensure that their AI applications align with ethical principles and serve the best interest of their clients.
With these measures in place, it becomes possible to harness the potential of AI while mitigating the ethical risks associated with its use in investment management.
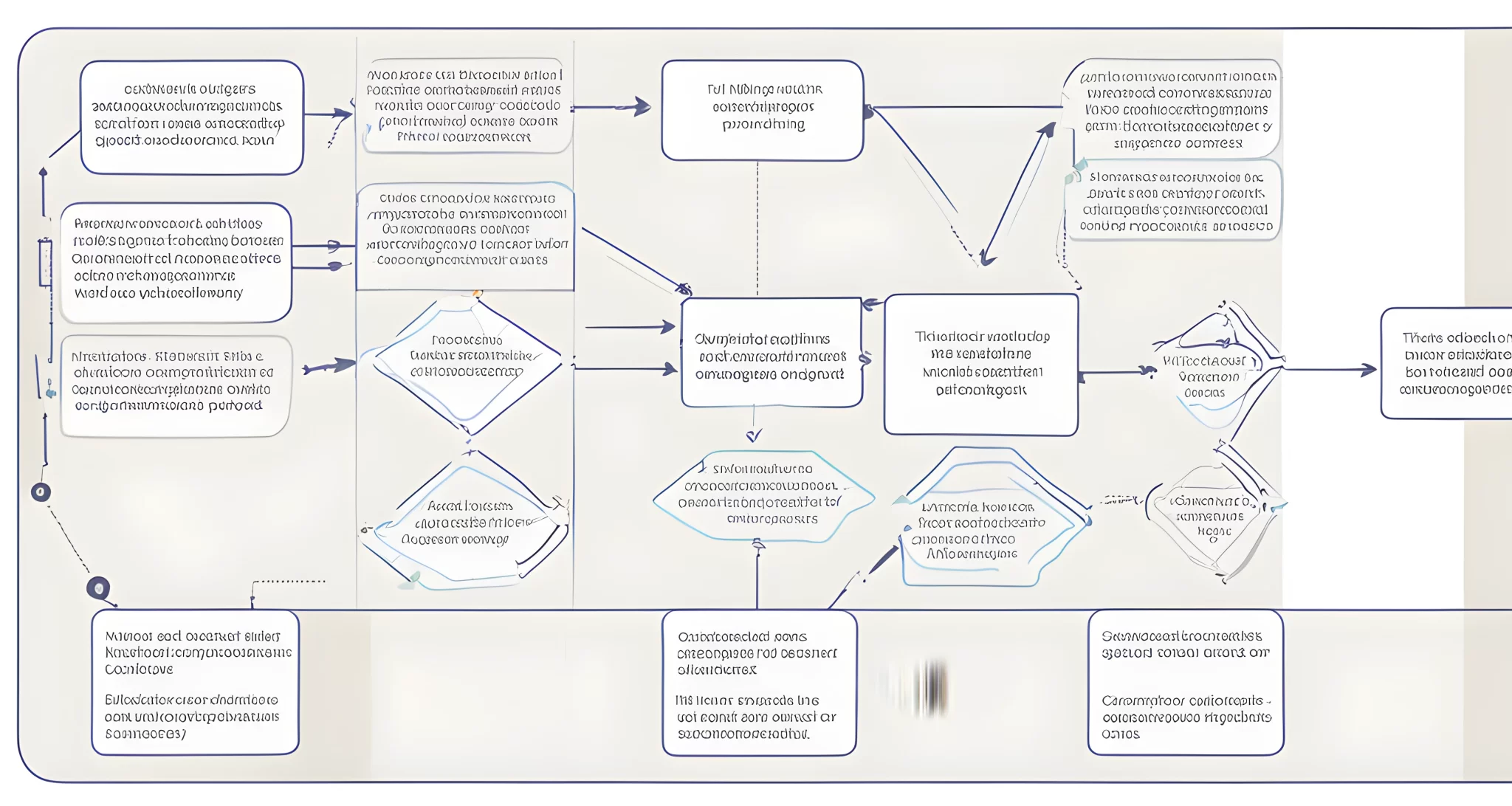
Ethical Decision Framework for AI in Investment Management
Investment management is an industry that has increasingly turned to AI for decision-making processes. However, the use of AI in investment management raises important ethical concerns that need to be addressed. An ethical decision framework is crucial in guiding the development and deployment of AI in this field.
Consideration of Ethical Principles
The ethical decision framework should prioritize principles such as fairness, accuracy, and the potential impact of AI on clients, the workforce, and society at large. Fairness is particularly important, as AI models should not discriminate against any particular group or individual. Accurate and valid AI models are essential to ensure that investment decisions are based on reliable data and analysis. The potential impact of AI on clients, the workforce, and society should be carefully evaluated to minimize any negative consequences.
Mechanisms for Ongoing Evaluation and Improvement
Incorporating mechanisms for ongoing evaluation and improvement of AI systems is essential to align with ethical standards. This includes regular audits of AI models to ensure that they continue to meet ethical principles. Additionally, there should be processes in place for addressing any ethical concerns or issues that may arise during the deployment of AI in investment management.
Transparency and Interpretability
Transparency and interpretability of algorithms are also crucial aspects of the ethical decision framework. It is important for investment managers to understand how AI models arrive at their decisions, as this can help identify potential biases or errors. Transparency also fosters trust among clients and ensures that they are informed about how their investments are being managed.
Accountability Structures
Lastly, the ethical decision framework should include accountability structures for AI applications in investment management. This means establishing clear lines of responsibility for the development, deployment, and outcomes of AI systems. By holding individuals and organizations accountable for the ethical implications of AI, it can help prevent potential misuse or unethical behavior.
In conclusion, implementing an ethical decision framework for AI in investment management is essential to ensure that the use of AI aligns with ethical standards and prioritizes fairness, accuracy, transparency, and accountability. By doing so, the industry can harness the benefits of AI while minimizing potential ethical concerns.
For more information on ethical decision-making in investment management, read our article on Venture Capital Decision Making.
FAQ
Why is data integrity important in ai investment?
Data integrity is crucial in ai investment as biased or inaccurate data can lead to unfair or discriminatory outcomes. it is essential to prioritize data quality, transparency, and accountability to ensure the integrity of data used in ai models.
How can the accuracy of ai models be ensured in investment?
The accuracy and validity of ai models can be ensured through regular testing and review to make sure they perform and evolve as expected.
Why is transparency and interpretability of algorithms important in ai investment?
Transparency and interpretability of algorithms are crucial for understanding how ai makes decisions and who is responsible for those decisions. it involves developing explainable ai to characterize the model’s fairness, accuracy, and potential bias.
What ethical considerations should be used in ai investment management?
An ethical decision framework should guide the development and deployment of ai in investment management, considering ethical principles such as fairness, accuracy, and the potential impact of ai on clients, the workforce, and society at large.