Challenges in the Financial Industry
One of the primary challenges in integrating artificial intelligence (AI) into the financial industry is the requirement for high-quality data. This is because financial decisions often depend on large and complex datasets, and AI algorithms need accurate and reliable data to make effective predictions and decisions. Without high-quality data, the potential benefits of AI in finance cannot be fully realized.
Furthermore, the financial industry is heavily regulated, and there is a need for transparency and explainability in AI models. This is crucial because decisions made by algorithms can have significant consequences for individuals and businesses. Therefore, ensuring that AI models are compliant with regulations and provide clear explanations for their decisions is essential for gaining trust and acceptance in the finance sector.
In addition to these challenges, there is also the issue of funding AI initiatives in the financial industry. Startups that are developing AI technologies for finance often face difficulties in securing funding to support their research and development efforts. However, there are opportunities for funding through venture capital firms that specialize in supporting AI startups in various industries, including finance. For more information on funding AI startups with venture capital, you can read the article Funding AI Startups.
Overall, while there are many opportunities to leverage AI in finance, such as improving risk management, fraud detection, and customer service, there are also significant challenges that need to be addressed. Overcoming these challenges will require collaboration between technology experts, financial professionals, regulators, and investors to ensure that AI can be effectively integrated into the financial industry while meeting regulatory standards and ethical considerations.
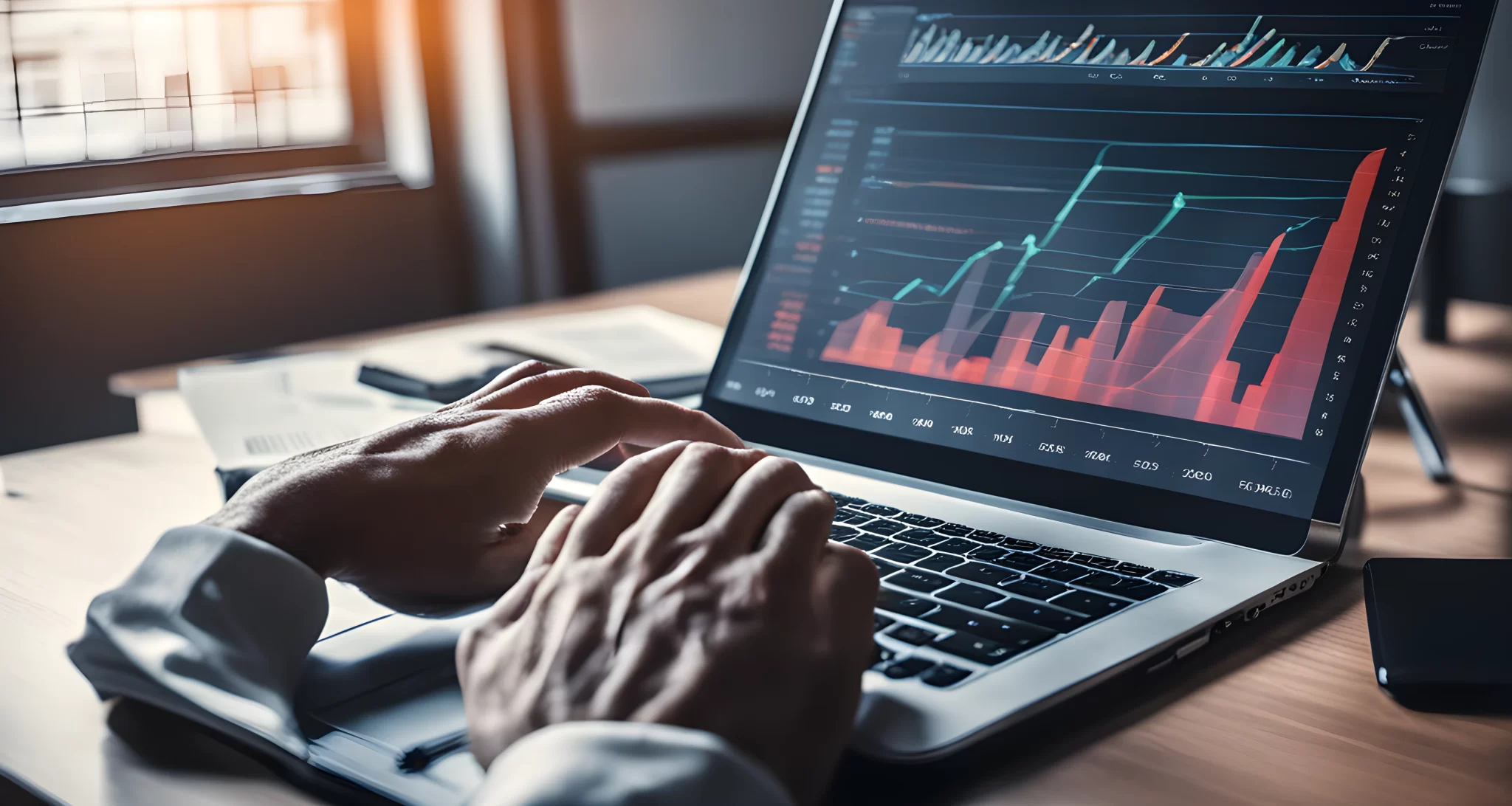
Opportunities in Finance with AI
Despite the challenges, AI offers numerous opportunities in finance. One significant application is credit scoring, where machine learning algorithms accurately assess the creditworthiness of individuals and businesses. AI is also extensively used for fraud prevention, as it can identify patterns and anomalies indicating fraudulent activity. Furthermore, AI is increasingly applied in algorithmic trading, analyzing market data in real-time and making split-second decisions to maximize profits.
The use of AI in finance has revolutionized the industry, allowing for more efficient and accurate processes. According to Finance Revolutionized by AI, the use of AI techniques has led to significant advancements in areas such as risk management, customer service, and investment strategies. With AI, financial institutions can streamline their operations, reduce costs, and provide better services to their clients.
The ability of AI to analyze vast amounts of data and identify patterns has also led to improved risk management practices. By utilizing machine learning algorithms, financial institutions can better assess potential risks and make more informed decisions. This has ultimately led to a more stable and resilient financial industry.
Moreover, AI has also opened up new avenues for investment strategies. With real-time analysis of market data and the ability to make split-second decisions, algorithmic trading has become more sophisticated and profitable. This has allowed for the development of new investment products and strategies that were not previously possible.
Overall, the opportunities presented by AI in finance are vast and continue to grow as technology advances. Financial institutions that embrace AI have the potential to revolutionize their operations and provide better services to their clients. As regulations and transparency in AI models continue to evolve, the future of AI in finance looks promising.
- Credit scoring
- Fraud prevention
- Algorithmic trading
- Risk management
- Real-time analysis of market data
- Investment strategies
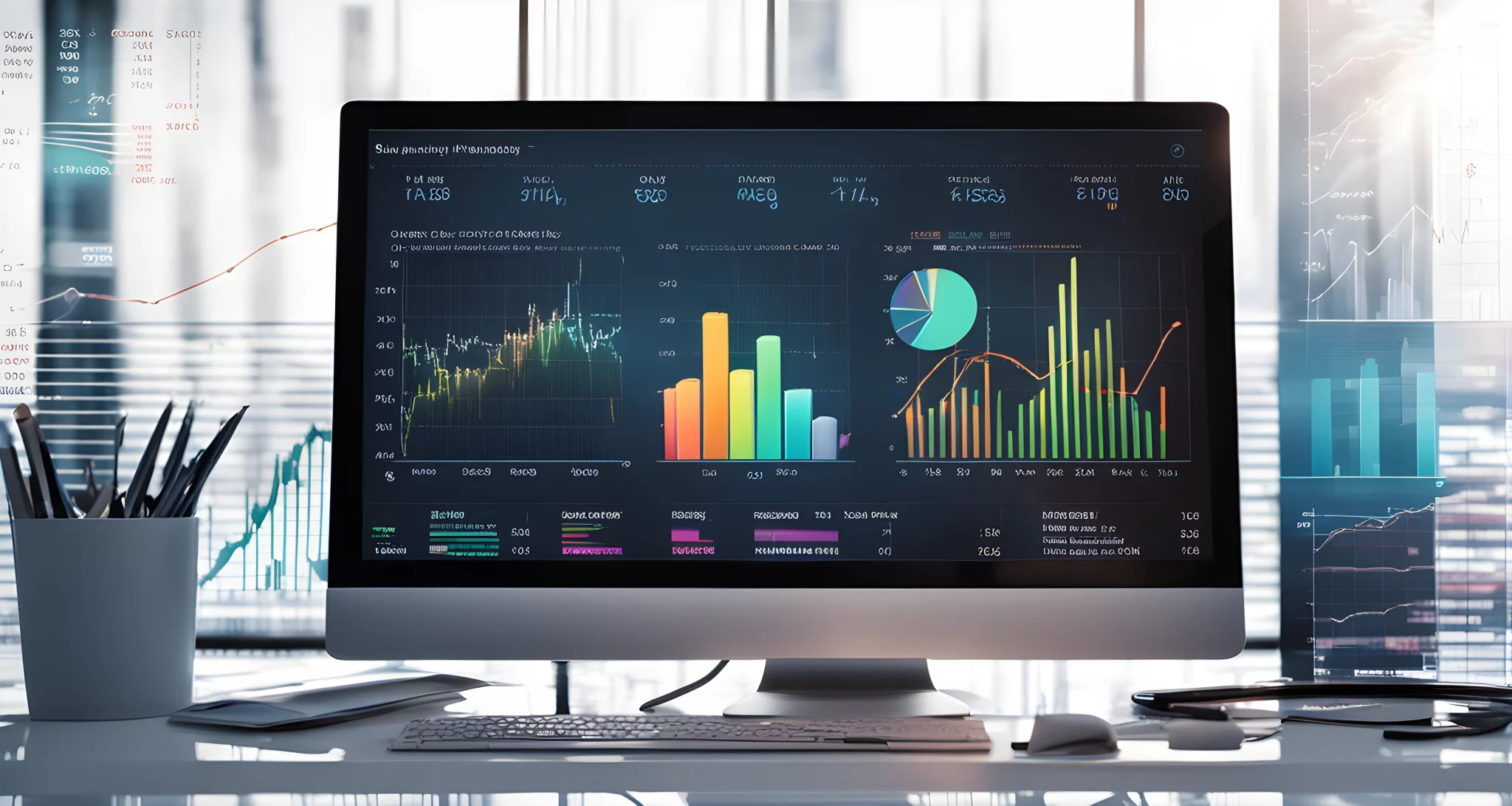
Use of AI Techniques in Finance
The use of AI techniques in finance is becoming increasingly prevalent as businesses look to leverage the power of artificial intelligence to gain a competitive edge. Both classic and modern AI techniques are utilized in finance, offering a range of benefits and capabilities.
Traditional techniques such as logistic regression and decision trees are preferred for their simplicity and explainability. These methods have been used for decades in finance to make predictions and inform decision-making processes. Their transparency and ease of implementation make them attractive options for financial institutions seeking to integrate AI into their operations.
On the other hand, modern techniques such as deep learning and neural networks offer more advanced capabilities, allowing for complex pattern recognition and analysis. However, these methods can be more complex to implement and require large amounts of data to train effectively.
The combination of both classic and modern AI techniques allows financial institutions to harness the power of AI in a way that suits their specific needs. Whether it’s using logistic regression to analyze customer behavior or implementing deep learning algorithms to detect fraudulent activity, AI offers a diverse range of applications in the finance industry.
For more insights into the potential of AI in finance, you can explore the article AI Venture Funding Potential, which delves into the opportunities that venture capital presents for the development and implementation of AI technologies in the financial sector. This article provides valuable insights into how AI techniques are being embraced by investors and businesses within the finance industry.
As technology continues to advance, we can expect AI techniques to play an increasingly important role in shaping the future of finance. From risk assessment and portfolio management to customer service and fraud detection, the possibilities for AI in finance are vast, providing numerous opportunities for innovation and growth within the industry.
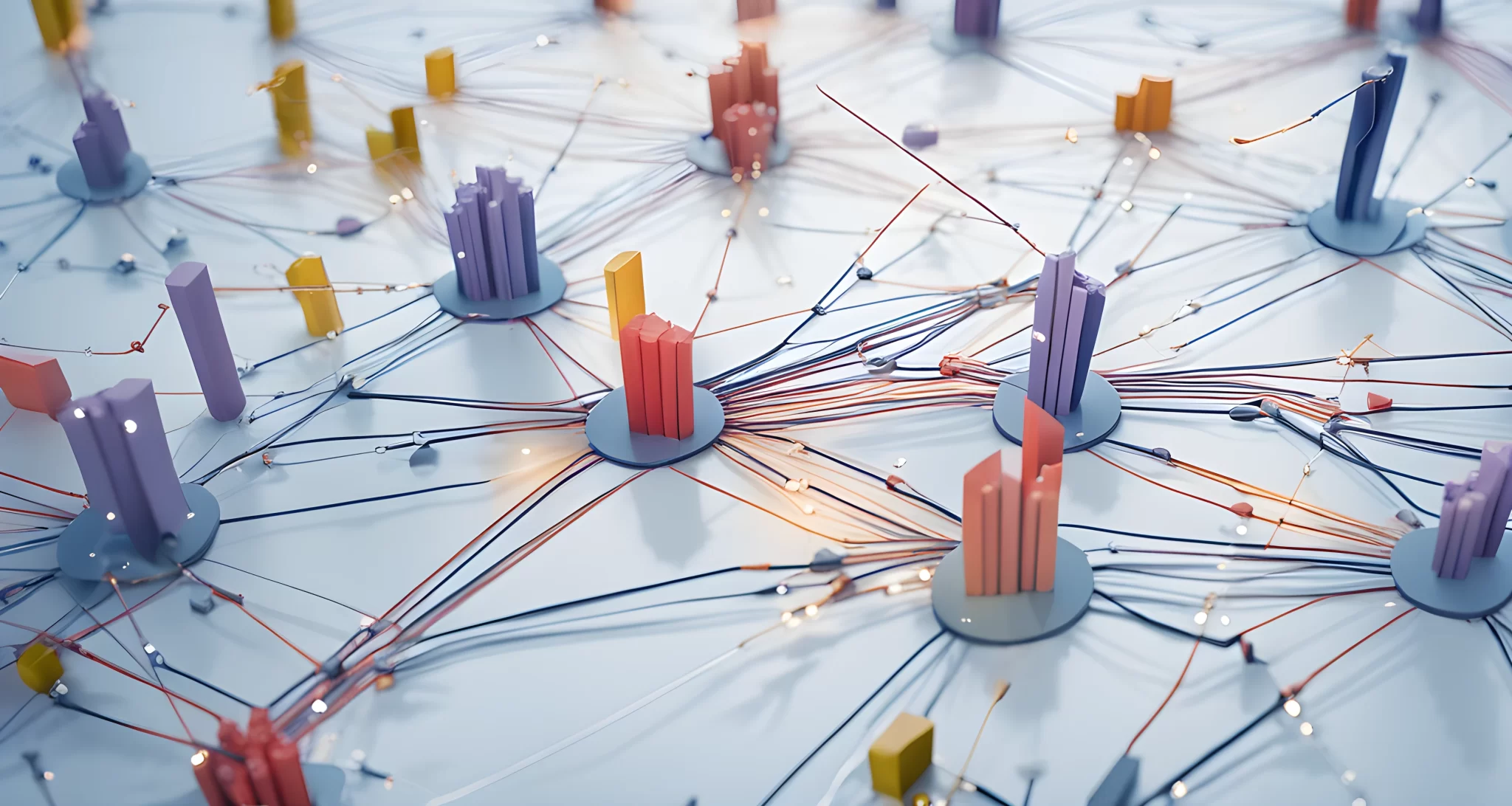
Significance of High-Quality Data in AI
The need for high-quality data in AI cannot be understated in the financial industry. Financial decisions rely heavily on accurate and reliable data, and AI algorithms require such data to make effective predictions and decisions. This emphasizes the importance of obtaining and maintaining high-quality data for successful implementation of AI in finance.
In today’s digital age, the amount of data available is unprecedented, but the quality of this data is what truly matters when it comes to AI in finance. High-quality data ensures that AI models can make informed decisions, leading to better risk management, investment strategies, and customer service in the financial sector.
The use of AI in finance has brought about numerous opportunities, but it also comes with its set of challenges. One such challenge is ensuring that the data used by AI systems is accurate and reliable. Without high-quality data, the effectiveness of AI algorithms is compromised, potentially leading to erroneous predictions and decisions with significant financial implications.
To address this challenge, financial institutions are investing heavily in data management processes that prioritize data quality. This includes implementing robust data validation techniques, leveraging advanced analytics tools, and establishing stringent data governance frameworks. Moreover, these institutions are also increasingly partnering with specialized data providers to ensure access to high-quality financial data.
Furthermore, regulations and transparency in AI models play a crucial role in upholding the quality of data used in finance. Regulatory bodies are actively involved in overseeing the use of AI in finance and are emphasizing the importance of using high-quality data for AI applications.
Overall, high-quality data is fundamental for the success of AI in finance. Financial institutions need to recognize the significance of investing in robust data management processes and ensuring compliance with regulations to harness the full potential of AI technologies. With access to reliable and accurate data, AI can revolutionize the financial industry by driving smarter decision-making, enhancing customer experiences, and optimizing operational efficiencies.
For more information on how AI is being used in different industries including startups, check out Startup AI Usage Today.
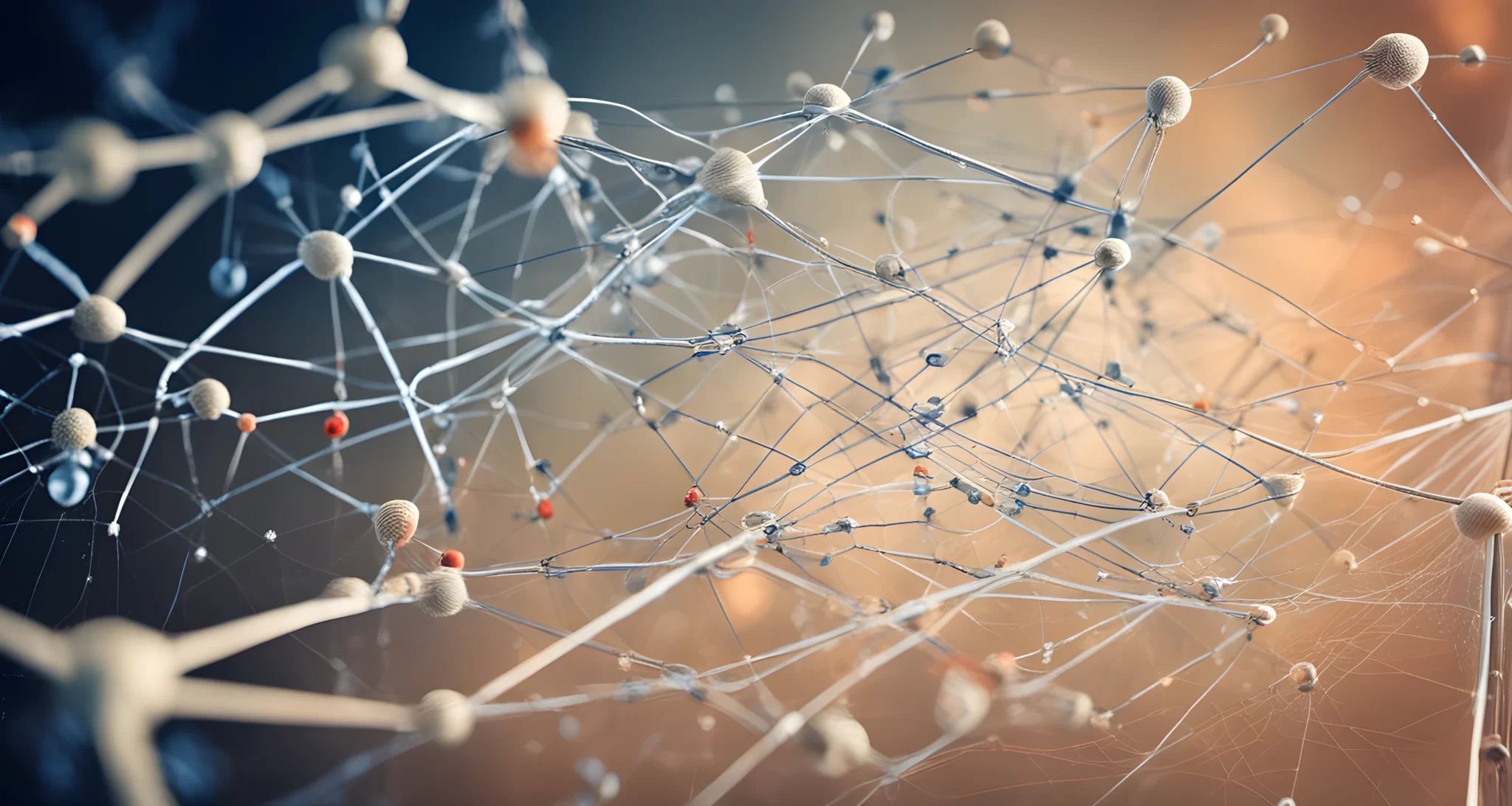
Regulations and Transparency in AI Models
In the financial industry, regulations and transparency are crucial in AI models. There is a need for transparency and explainability in AI models, as decisions made by algorithms can have significant consequences for individuals and businesses. The heavily regulated nature of the financial industry further emphasizes the importance of complying with regulations and maintaining transparency in AI models.
Regulatory bodies such as the Securities and Exchange Commission (SEC) and the Financial Industry Regulatory Authority (FINRA) have strict guidelines in place to ensure that AI models are developed and deployed responsibly. These regulations aim to protect consumers and ensure fair practices within the industry. As a result, financial institutions must adhere to these regulations to avoid legal repercussions.
Transparency in AI models is also essential for building trust with both customers and regulators. By providing clear explanations of how AI algorithms make decisions, financial institutions can demonstrate accountability and fairness. This transparency not only helps to mitigate potential risks but also fosters confidence in the use of AI within the industry.
Furthermore, AI Integration Challenges require financial institutions to carefully evaluate and document their AI models to ensure compliance with regulations. This includes providing detailed documentation on model development, data sources, algorithmic choices, and testing procedures. By doing so, financial organizations can demonstrate that their AI models are ethical, unbiased, and compliant with regulatory standards.
In conclusion, regulations and transparency play a critical role in the development and deployment of AI models within the financial industry. Adhering to regulatory guidelines and maintaining transparency not only fosters trust but also ensures that AI is used responsibly to benefit both businesses and consumers.
Overall word count: 248
FAQ
What are the primary challenges of ai in the financial industry?
The primary challenges include the need for high-quality data, heavy regulation, and the requirement for transparency and explainability in ai models due to the significant consequences of algorithmic decisions.
What are the opportunities of ai in finance?
Ai offers opportunities in credit scoring, fraud prevention, and algorithmic trading, allowing for accurate assessment of creditworthiness, identification of fraudulent activity, and real-time analysis of market data for profit maximization.
What techniques are commonly used in ai for finance?
Both classic techniques like logistic regression and decision trees, as well as modern techniques like deep learning and neural networks, are used in finance. classic techniques are preferred for their simplicity and explainability, while modern techniques offer more advanced capabilities.
Are the challenges worth the opportunities in ai for finance?
Despite the challenges, the opportunities offered by ai in finance are significant, making the implementation of ai in the financial industry worthwhile for the potential benefits it brings.