Understanding AI Risks
When it comes to managing AI risks for businesses, it is essential to have a comprehensive understanding of the potential challenges and drawbacks that can arise. Prioritizing ethical considerations, conducting thorough risk assessments, and implementing robust security measures are all crucial components of this process.
One of the key risks associated with AI is the potential for inaccuracies in the data and algorithms used to power AI systems. This can lead to incorrect or misleading results, which can have serious implications for businesses. Additionally, safety concerns must be addressed, particularly in industries where AI is used to make critical decisions or operate machinery.
Dishonesty is another significant risk that businesses must consider. AI systems can be vulnerable to manipulation or bias, which can undermine their reliability and trustworthiness. Lack of empowerment and sustainability are also important risks to manage, as AI has the potential to displace human workers and contribute to environmental degradation if not carefully regulated.
To mitigate these risks, businesses should follow best practices such as using zero or first-party data, keeping data fresh and well-labeled, ensuring there’s a human in the loop AI return on investment, testing and re-testing AI systems, and seeking feedback from users and stakeholders. By taking these steps, organizations can minimize the potential negative impacts of AI and ensure that their use of this technology is ethical, reliable, and secure.
In conclusion, understanding AI risks is a critical aspect of managing AI for businesses. By prioritizing ethical considerations, conducting thorough risk assessments, and implementing robust security measures, organizations can mitigate the potential challenges associated with generative AI. By following best practices for managing AI risks, businesses can harness the power of AI while minimizing its potential drawbacks.
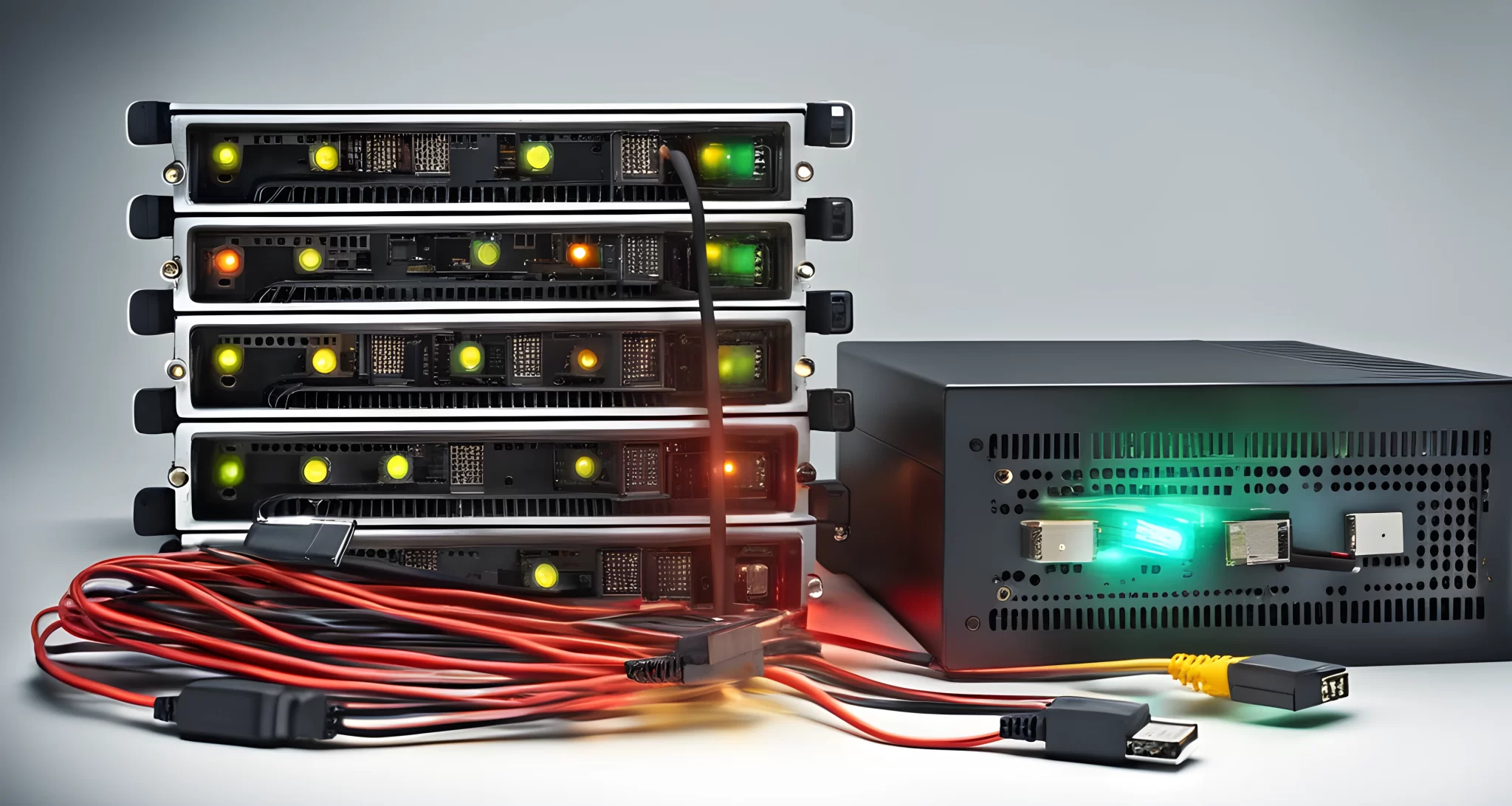
Mitigating Risks with Data
When it comes to managing AI risks, one of the key strategies businesses can employ is mitigating risks with data. This involves prioritizing the use of zero or first-party data, keeping data fresh and well-labeled, ensuring there’s a human in the loop, testing and re-testing, and getting feedback.
Prioritizing zero or first-party data means relying on data that is collected directly from your own customers or operations, rather than third-party sources. This helps to ensure the integrity and accuracy of the data being used for AI applications.
Keeping data fresh and well-labeled is essential for effective risk mitigation. Fresh data ensures that AI models are trained on the most current information, while well-labeled data helps to avoid bias and errors in the AI system.
Ensuring there’s a human in the loop means having human oversight and intervention in AI processes. This can help catch errors or biases that may not be apparent to the AI system alone.
Testing and re-testing AI systems is crucial for identifying and addressing potential risks. Regular testing helps to uncover any issues or vulnerabilities before they become major problems.
Getting feedback from users and stakeholders can provide valuable insights into how AI systems are functioning and where potential risks may lie.
In addition to these measures, businesses should also undertake a thorough AI risk assessment. This involves evaluating the integrity of training datasets, probing the resilience of security measures, and unmasking hidden vulnerabilities and unanticipated consequences. By taking these steps, businesses can better protect themselves from potential AI risks.
For more information on effective risk management strategies for consulting firms, check out Effective consulting risk solutions.
By prioritizing the use of quality data and implementing robust risk management strategies, businesses can significantly reduce the potential negative impacts of AI on their operations.
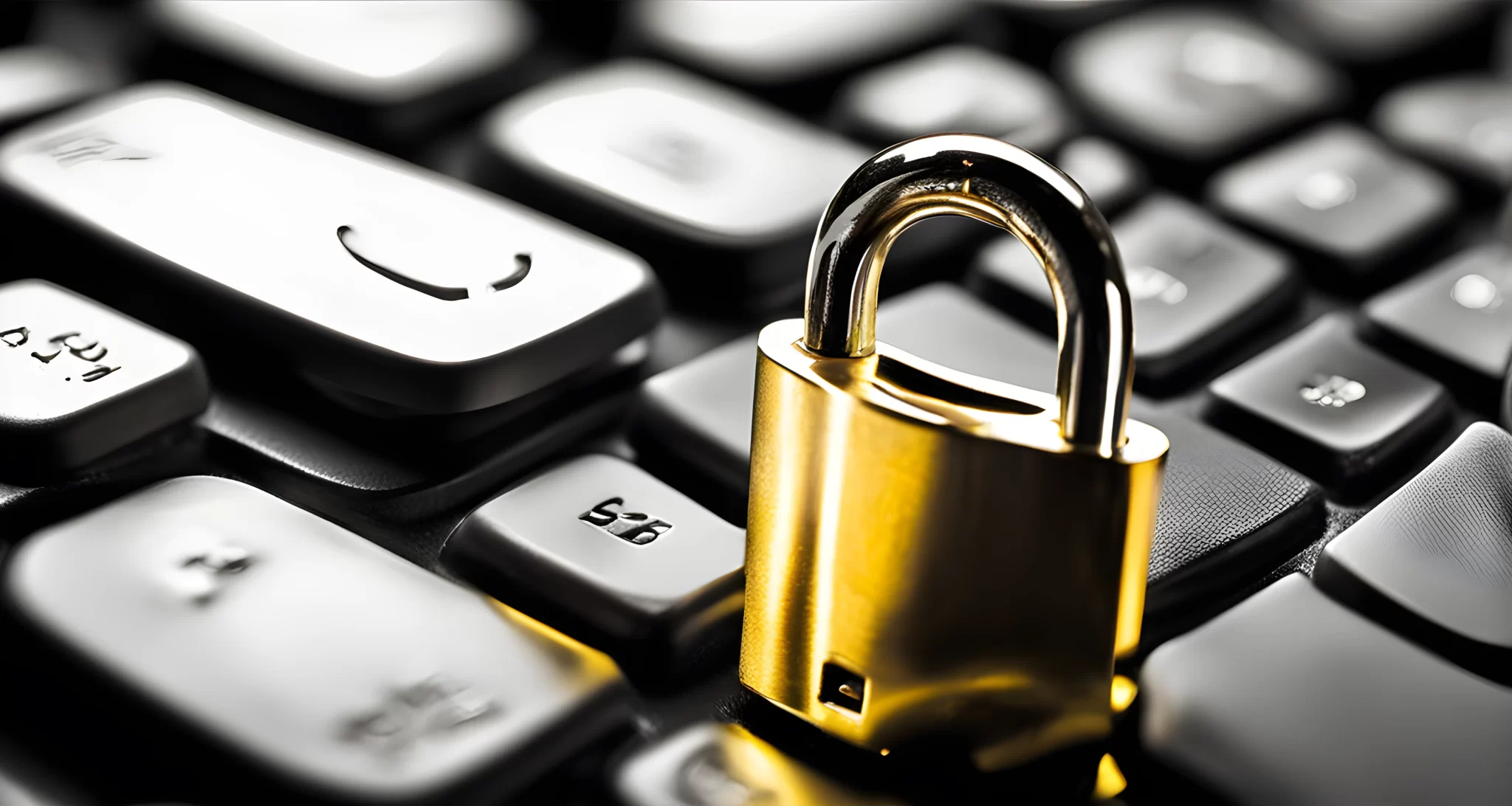
Implementing Robust Security Measures
When it comes to managing the risks associated with AI, implementing robust security measures is crucial. These measures are necessary to protect against potential threats and ensure the safe and reliable operation of AI systems.
Incident Response Plans
One of the key components of robust security measures is the development and implementation of incident response plans. These plans outline the steps to be taken in the event of a security breach or other related incidents. By having a well-defined response plan in place, organizations can minimize the impact of potential security threats and mitigate any damages that may occur.
Dynamic Approach
In addition to incident response plans, it is essential for organizations to adopt a dynamic approach to security. This means staying abreast of the evolving nature of AI and continuously updating security measures to address new threats. By staying proactive and adaptable, organizations can better safeguard their AI systems against emerging risks.
Workplace Risks
It’s also important for organizations to be aware of the workplace risks associated with AI. This includes potential job displacement, biased recruitment practices, privacy concerns, and lack of implementation traceability. By taking these risks into account, organizations can better address security measures that mitigate these potential issues.
In conclusion, implementing robust security measures is essential for managing AI risks effectively. By developing incident response plans, adopting a dynamic approach to security, and addressing workplace risks, organizations can better protect their AI systems and ensure their safe and reliable operation.
To learn more about the importance of risk management in business, check out Risk management for business success.
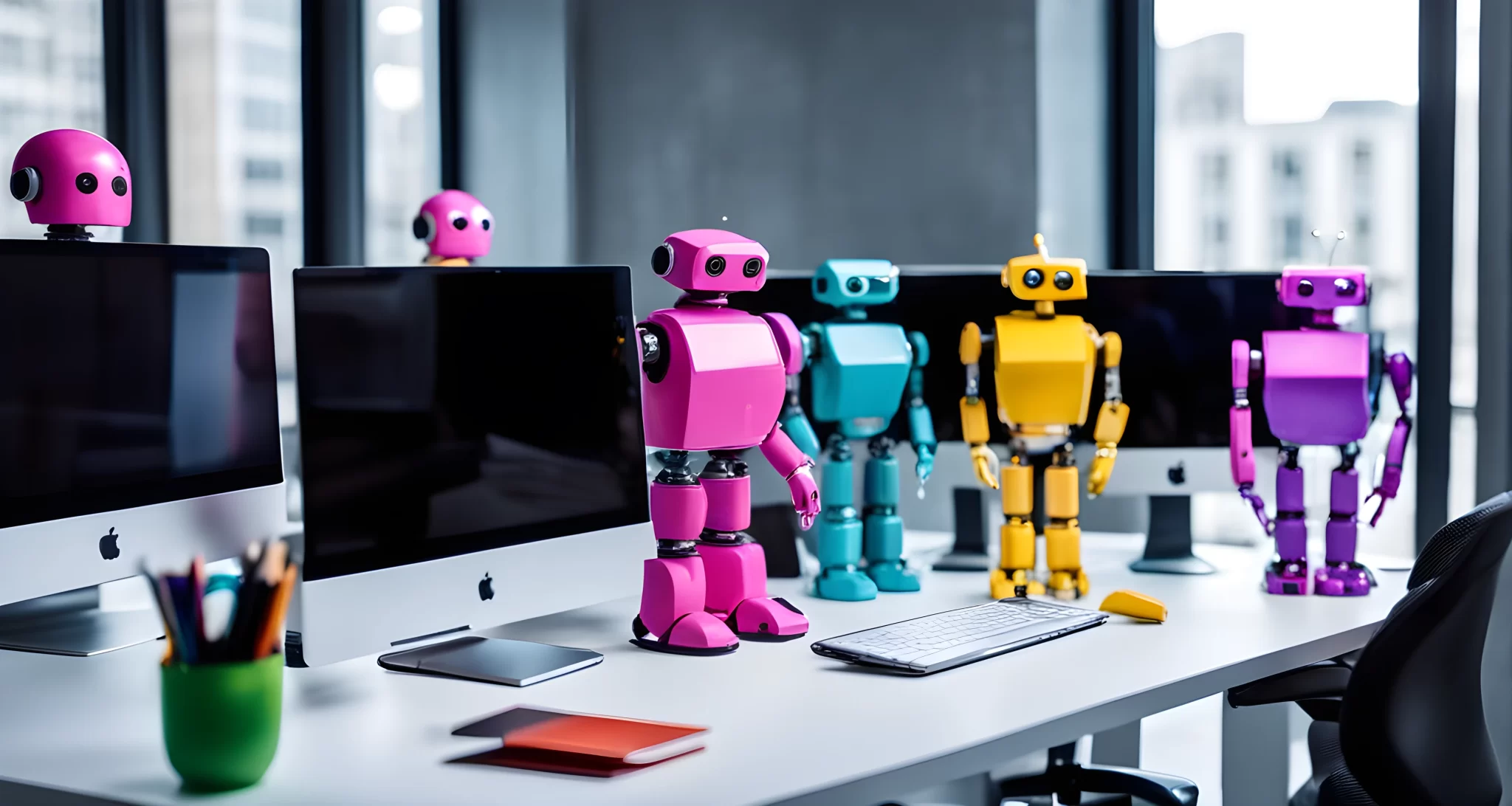
Addressing Workplace Risks
Addressing Workplace Risks:
Workplace risks associated with AI, such as job displacement, biased recruitment practices, privacy concerns, and lack of implementation traceability, require a multidisciplinary approach involving leaders in the C-suite and across the company, experts in various fields, and managers at the front lines to address. Thorough AI risk assessment is also necessary, evaluating the integrity of training data sets, probing the resilience of security measures, and unmasking hidden vulnerabilities and unanticipated consequences.
To effectively address workplace risks associated with AI, businesses must take proactive measures to mitigate potential negative impacts. This involves implementing robust strategies to ensure fair and equitable treatment of employees and candidates in all AI-related processes. Additionally, companies should prioritize data privacy and security to protect sensitive information from unauthorized access or misuse. By doing so, businesses can build trust among employees and customers while also complying with regulatory requirements.
Furthermore, it is crucial for businesses to establish clear accountability and transparency in their AI implementation. This includes creating mechanisms for tracking and tracing the decision-making process of AI systems to identify potential biases or errors. Implementing clear oversight and governance structures can help minimize the risk of unintended consequences or discriminatory practices.
In addition to internal measures, businesses should also stay informed about the latest developments in AI technology and their potential impact on the workforce. Engaging with industry experts and participating in forums such as AI in Finance can provide valuable insights for businesses looking to stay ahead of the curve and adapt their strategies to evolving trends.
By taking a comprehensive approach to addressing workplace risks associated with AI, businesses can create a safer and more inclusive environment for their employees while leveraging the benefits of this transformative technology.
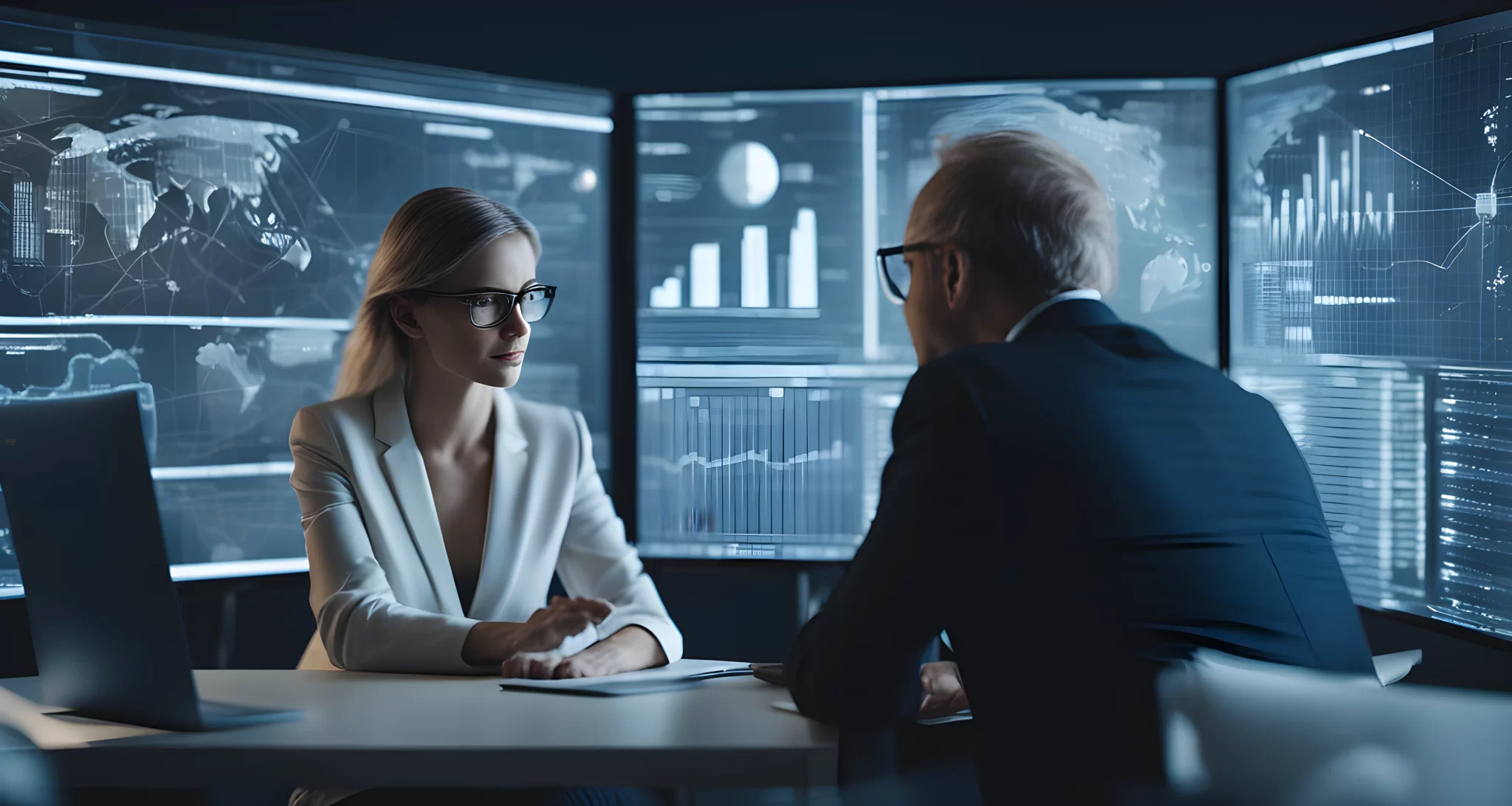
Best Practices for Managing AI Risks
When it comes to managing AI risks, organizations need to adopt best practices that prioritize ethical considerations, risk assessment, and robust security measures. Here are some key best practices to consider:
-
Prioritize the use of zero or first-party data: Utilizing data that is collected directly from the source or from trusted partners can help mitigate the risks associated with using third-party data, which may be unreliable or unethical.
-
Keep data fresh and well-labeled: Ensuring that data used for AI models is up-to-date and accurately labeled can help minimize the potential for biased or inaccurate outcomes.
-
Ensure there’s a human in the loop: Having human oversight and intervention in AI processes can help catch errors, biases, and ethical concerns before they escalate.
-
Test and re-test: Continuously testing AI models for accuracy, fairness, and robustness is crucial for identifying and addressing potential risks before they cause harm.
-
Get feedback: Soliciting feedback from stakeholders, employees, and end-users can provide valuable insights into potential risks and issues that may not have been initially considered.
-
Implement robust security measures: In addition to considering ethical and data-related risks, organizations must also prioritize the implementation of strong security measures to protect against cyber threats Dealing with Risk is Important. This includes having incident response plans in place to address potential workplace risks associated with AI.
A multidisciplinary approach involving leaders in the C-suite and across the company, experts in various fields, and managers at the front lines is also necessary to effectively manage AI risks. By bringing together diverse perspectives and expertise, organizations can better identify, assess, and address potential risks associated with AI implementation.
Overall, managing AI risks requires a comprehensive approach that takes into account ethical considerations, data quality, human oversight, continuous testing and feedback, as well as robust security measures. By prioritizing these best practices, organizations can navigate the complexities of AI while minimizing potential risks.
FAQ
What are the potential risks associated with generative ai?
The potential risks associated with generative ai include inaccuracy, safety concerns, dishonesty, lack of empowerment, and sustainability issues.
What are some best practices for managing ai risks?
Some best practices for managing ai risks include using zero or first-party data, keeping data fresh and well-labeled, ensuring there’s a human in the loop, testing and re-testing, and getting feedback.
How can organizations address workplace risks associated with ai?
Organizations can address workplace risks associated with ai by taking a multidisciplinary approach involving leaders in the c-suite and across the company, experts in various fields, and managers at the front lines.
What should be included in a thorough ai risk assessment?
A thorough ai risk assessment should involve evaluating the integrity of training data sets, probing the resilience of security measures, and unmasking hidden vulnerabilities and unanticipated consequences.